Paper-Weekly18-The Flan Collection: Designing Data and Methods for Effective Instruction Tuning
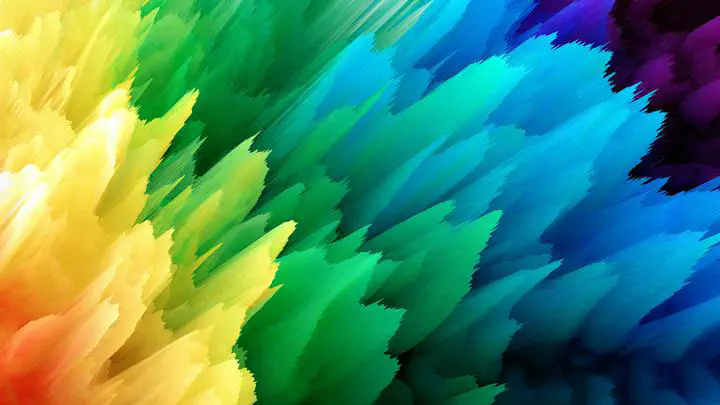
We find task balancing and enrichment techniques are overlooked but critical to effective instruction tuning, and in particular, training with mixed prompt settings (zero-shot, few-shot, and chain-of-thought) actually yields stronger (2%+) performance in all settings.

instruction tuning 经典数据集,主要结论如下:
- 混合zero-shot和few-shot yields better results.
- 验证一些trick的有效性:scaling, enriching task variety with input inversion, adding CoT, balancing different data source
- Demonstrate these technical choices yield 3-17% Held-Out task improvements over existing open source instruction tuning collections
- Demonstrate Flan-T5 serves as a stronger and more computationally-efficient starting checkpoint for single-task finetuning

实际上这篇文章写得过于清楚以至于看这几个图表就够了

比较反直觉的是把0-shot和few-shot数据混合后,在两者的eval上都取得了更好的效果。或者现在看来这也不反直觉,毕竟无论是哪种setting, training data一定是不够的。

把任务数量scale up后,在参数量更大的模型上更早达到best performance;held-in task已经出现了overfit,但是held-out task上还underfit。

把instruction tune过的t5在specific dataset上finetune的表现也比vanilla T5收敛的更快

这篇文章的related work也写的非常好,赞一个。